Sylvain Lobry
Université Paris Cité, LIPADE laboratory, SIP team.
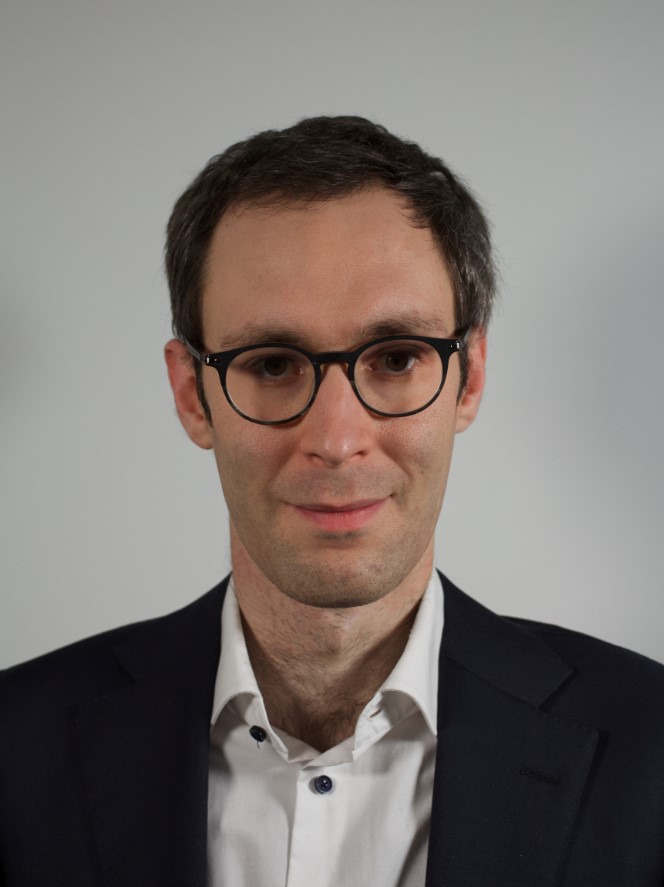
Bureau 812H
45, rue des Saints-Pères
75006 Paris France
Email: firstname.lastname@u-paris.fr
I am an assistant professor (Maître de conférences) in Computer Science. I do research at the SIP team of the LIPADE laboratory and teach at UFR de Mathématiques et Informatique in Université Paris Cité. Before, I was a postdoctoral researcher at Wageningen University & Research in the Laboratory of Geo-information Science and Remote Sensing. I obtained my PhD in image processing from Télécom Paris in 2017. This work was done in collaboration with CNES.
My research interests are in the areas of methodological developments in image processing with applications in particular to remote sensing imagery. This includes high-resolution optical images processing using deep learning techniques and change detection, classification and regularization on multi-temporal series of SAR images using Markov Random Fields models. During my PhD, I was working on the SWOT mission, dedicated to the study of the world’s oceans and its terrestrial surface waters.
News
Apr 11, 2024 | Nathan successfully defended his PhD today. Congrats Nathan! |
---|---|
Mar 30, 2024 | I will give a talk at G2AS’24 on April 17. |
Mar 1, 2024 | Nicolas Houdré is starting his MSc internship with us today! |
Feb 5, 2024 | New special issue in ISPRS Photo |
Feb 1, 2024 | Yuxing Chen is joining us as a Post-doctoral student today! Welcome! |
Jan 1, 2024 | Swann Briand is starting his PhD today! |
Dec 7, 2023 |
|
Nov 29, 2023 |
|
Nov 22, 2023 | New DiiP strategic project with LISA to work on climate monitoring from RS data! |
Nov 13, 2023 | I will be at Dagstuhl for the Space and AI seminar. |